Learning versus monitoring and evaluation
There has been a large body of knowledge developed in recent years on Monitoring, Evaluation, and Learning (MEL), especially in the international development sector. Typically, these MEL schemes have focused on accountability, which means demonstrating to donors and others that resources have been spent appropriately. While this is obviously an important activity, it does not necessarily lead to deeper learning and will not really help you to navigate towards more sustainable futures.
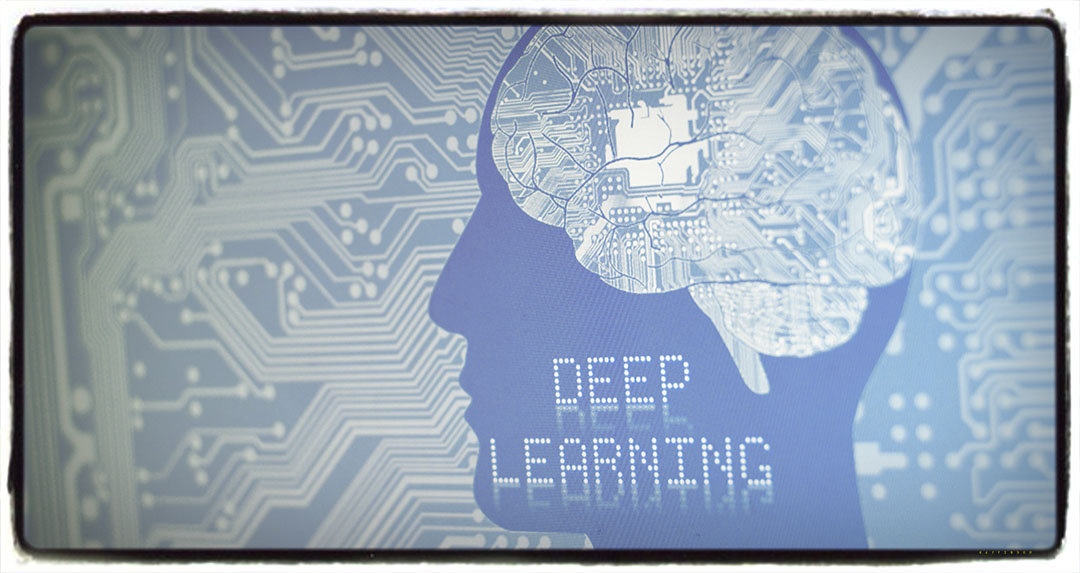
When working with complex systems, deep learning approaches are needed to question and challenge your underlying assumptions. Within this type of framework any specific kind of monitoring an evaluation can be developed. Photo: iStock.
Rather than starting to develop specific indicators for success, the most critical task is actually to establish a learning framework that enables deep learning. Within this framework any specific monitoring scheme can be developed later but starting with a learning framework means you are likely to ask the right questions at the right level for the right reasons, rather than just developing sets of indicators and measures that may not drive real learning and change. See the attached case from AWARD in South Africa, an organization that uses a deep learning approach in their projects to tackle issues of sustainability, inequity and poverty.
Different levels of learning
Learning can occur at many levels. Basic learning, or “single-loop learning”, can come from simply asking questions about the expected outcomes from an action. For example, in a project to restore fish populations in a river, after a series of interventions you may ask by how much the numbers of fish increased. This level of learning is useful for assessing the efficiency and/or effectiveness of an action towards achieving an outcome in a context of certainty rather than uncertainty, but it is unlikely to drive deeper change in how people think about the river system. It is, for example, very unlikely that you capture any unintended consequences of the fish restoration activities.
When working with complex systems, a “double-loop” or “triple-loop” approach to learning is more appropriate. These types of approaches push you to deeper levels of enquiry; questioning and challenging your underlying assumptions. So, in the fish example above, following on from the initial evaluation questions about the relative success of achieving the increase in fish numbers, you might also ask questions about the broader consequences of your actions and the objectives of the project, for example:
- In addition to influencing fish stocks, what other effects did the intervention have?
- Why do we want to increase fish numbers?
- Who will benefit from increasing fish numbers?
- Who may be disadvantaged?
- What are the key assumptions we are making when we assume increasing fish numbers will improve food security for local people?
- What are the important assumptions we are making about the data and information relating to fish populations and the ecosystem?
These types of questions can then drive deeper reflections about the system, about the process of setting the objectives, and about the assumptions that were made at the time. This may drive more fundamental system change, reorganizing structures and changing power dynamics, and in some cases, transformative change can emerge. These kinds of triple-loop questions include, for example:
- Who set the objective of increasing fish populations?
- Was the process fair and transparent?
- Was it open to all stakeholders, including the less powerful or marginalized?
- How well does the decision-making process match the scale and dynamics of the system and issues?
- Is the long-term vision for the system a truly sustainable and fair one?
Making sure that you learn deeply
Setting up a learning framework that allows for this type of deeper enquiry, will force you to confront deeper issues about the underlying purpose, the structure and the function of your focal system. This type of framework will provide a good foundation for assessing if your action strategies continue to move you towards a sustainable, safe and just future in a highly uncertain and rapidly changing world (figure 36.1). Discuss with your Coalition and additional members of the Implementation team how you can develop such a framework to capture the outcomes of your implementation process.